A human approach to AI
Dr Djamila Amimer, CEO and Founder of Mind Senses Global, explores the potential of AI and automation and explains how, when used correctly, AI can transform your decision-making.
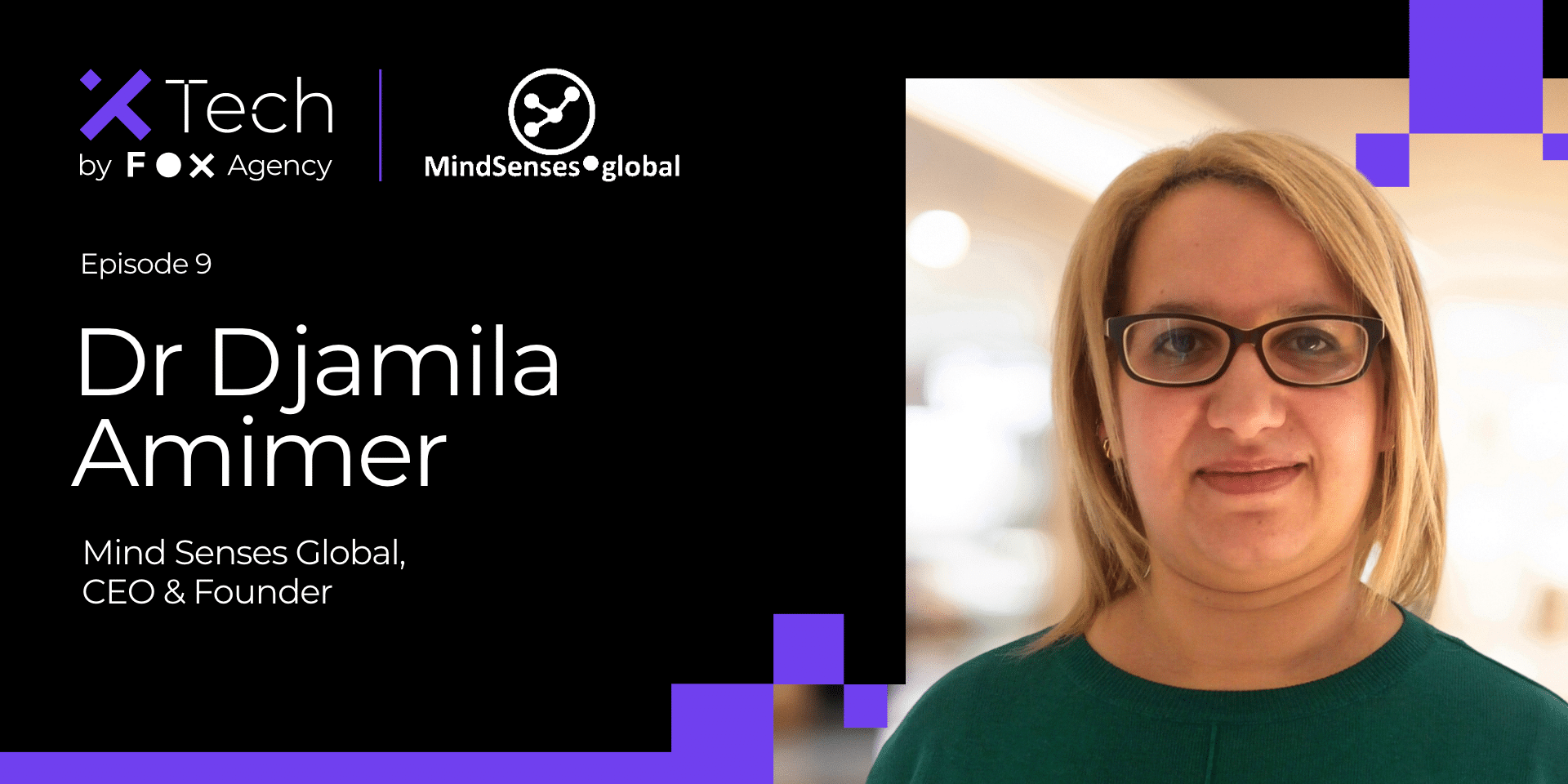
“If you are a business and you operate in a highly compliant sector, for example, financial services or healthcare, you really need to understand what the algorithms are doing.”
The secret behind AI success is a lot more than ‘plug and play’. In fact, the more you put in, the more you get out.
Dr Djamila Amimer, CEO and Founder of Mind Senses Global, shares her experience of helping businesses improve their decision-making using AI. This podcast explains what companies should be thinking about when implementing these powerful tools, and how to maximise investment.
Transcript:
Speaker 1:
Ready to explore the extraordinary world of tech. Welcome to the XTech Podcast where we connect you with the sharpest minds, leading voices in the global tech community. Join us as we cut through the complexity to give you a clear picture of the ideas, innovations, and insight that are shaping our future.
Debbie Forster:
Hello and welcome to the XTech Podcast by Fox Agency. I’m your host, Debbie Forster MBE. I’m the CEO at the Tech Talent Charter and an advocate and campaigner for diversity, inclusion, and innovation in the tech industry.
Today I’m joined by Dr. Djamila Amimer. She is the CEO and Founder of Mind Senses Global. Djamila, it’s lovely to have you here today.
Djamila Amimer:
Debbie, lovely to be with you. Thanks for the invitation.
Debbie Forster:
Djamila, one of the things that our community loves to hear is how a person gets into tech. Some go by a very straight route. They know from the very start that’s what they want to do. Others a more winding path. Tell me about yourself. How did you find yourself in tech?
Djamila Amimer:
That’s a great question, Debbie, because we all have different paths. So my journey started with mathematics. As a young child, I always loved mathematics. So I studied mathematics to the very advanced levels. Then at the university I studied operations research, which is applied mathematics to solve real business problem. So I found myself studying optimization techniques and simulations and Monte Carlo methods and so on. And then went into doing more advanced studies in my master degree where I started experimenting with physiologic and neural networks and genetic algorithms. So this is really the start of AI because those techs is what makes AI today. And then I finished that with the PhD in AI in economics. That was back in the early 2000s.
Back then it wasn’t very attractive to get a job in the tech industry or to get a job in AI. So started working in the energy sector. I worked for both BP and Shell, had several roles, commercial roles, business development roles. I worked in different divisions within the energy sector. Worked in upstream, in downstream, in shipping and trading. I got the chance and the opportunity to apply those AI techniques and algorithm to solve problem. We wouldn’t call it AI at that time, inside those companies, we will just see it as another tool. So it was quite an obvious option for me.
So when I decided to leave the energy sector in 2018 and start my own business, it was a natural move for me to set up a Mind senses Global, which is a boutique AI management consultancy. So my main priority is to help businesses and organization apply AI. And the way we do it is through education. I’m a great believer in educating people in the field of AI and making AI accessible to everyone. We also help with the AI strategy. Obviously, we have the tools to develop those AI platforms. But my advice to whoever I speak to in this field is you should never start with AI, you should start really with your business problem.
Debbie Forster:
I love the journey. So you were looking at AI from an academic perspective before it was a thing and before the business discovered it, and you’ve watched then since the noughties that go from something that felt like science fiction and something you read about by something like Azimov, to now that we’re really in the heart of the hype curve, aren’t we? You can’t swing a cat without finding something about AI, which is frustrating for, I think, some people in the field because when is it AI? When is it just great algorithms? When is it good machine learning? Et cetera. So let’s go back to a basic standpoint because not just explain to the audience many of whom may know it, but helping us understand a way to explain it to our wider business. What is and what isn’t AI?
Djamila Amimer:
Wow, that’s a great question because obviously because of the hype, everyone is claiming and defining AI the way that they see it. So my starting point, I really love the definition of John McCarthy. Actually, John McCarthy in 1956 was the first person who coined and basically used the term AI. And so let me refer to his definition. So the way he defined AI, he said “AI is the science and engineering of making intelligent machines.” Obviously, you know that from the definition it’s a little bit vague. You can have a lot of things in that. And that’s the critical bit to understand is that AI is not one thing. It’s a lot of things that comes under the umbrella of AI. So in AI we will find mathematics, we will find statistics, we will find neuroscience, we will find psychology, we will find ethics, and governance. So you have all those different fields that comes and make what we call the area of AI.
AI means a lot of things to different people. So for example, if you have a smartphone, then you are probably using AI, whether you know it or not. For some other people, AI means the drone technologies because they’re using those sophisticated techniques to have those flying machines. So that’s another piece of AI. For other people, AI is machine learning. So that’s the predictive ability. So this is the softwares that predict things for the business. This is also AI. It’s also computer vision, but it’s also picture. So if you ever come across what we call a fake picture, so this is really a not real picture. So it’s AI who created that picture. So that also is AI. Basically, it’s a lot of things. My rule of thumb whenever, okay, so how can we help people identify what is AI and it isn’t. My crucial rule is there a learning process happening in whatever application or methods.
So if the machine or the algorithm or the application is learning, and what I mean by that, it’s not like the way we human learn because the machine do not understand and we need to keep that in mind, but the learning in the sense that we are using past historical iteration or past historical data to make the next prediction better. And then once the machine gets the next prediction better, then again we are using that input to make then the next one better. So there is an iterative process, what we call learning process for the machine to make things better and better. Better in terms of accuracy, better in terms of the quality of the output and so on. So if there is a learning then it’s probably AI. If there is no learning and we’re not using any past historical iteration to make the future iteration better, then it’s not AI. And I think that’s a very simple rule that at least you can rule out what is not AI.
Debbie Forster:
And it’s really caught on in the last few months, even further in that hype curve on generative AI, ChatGPT. So the gold rush is on, we have a bunch of wild-eyed businesses running around wanting AI, not really sure what it is, how it is, how they’re going to put it to use. Let’s come back. If you’re working with a company and particularly you’re advising the techie people within that company, what should they be thinking about? Let’s pretend we’ve managed to educate our business owners to understand what AI is. Before we dive in and start looking at how we’re going to use AI within our company, what should we be thinking about?
Djamila Amimer:
As I mentioned, the starting point shouldn’t be AI, so it should be business, so whether it’s the business people or the techie people. And by the way, there are two school of thoughts. One school of thought that think that the techie or what they call the IT people should sit out in a separate division from the business people. There is another school that I belong to and specifically about the AI people. I really think that the AI and the data scientist people should sit in the business. They shouldn’t sit in what traditionally called IT services. So why? Because we really need to understand the business environment, the business context of that particular organization. We need to find in which area we are going to apply AI. We need to understand that AI can help in a lot of areas, there are a hundred ways you can use AI for your business.
So you can use AI to reduce your cost, you can use AI to increase your margins, you can use AI to reduce your risk, you can use AI to improve your customer experience or customer services. So you need to understand as a business having a solid business strategy, what are your aspiration, what you would like to achieve from a business perspective. Or another way to say it as a business, do you have currently issues that you need to sort out so you can grow in the future? So you have to identify, “I am going to use AI to improve my margins or to reduce my costs?” You have to prioritize. You cannot just try everything at once because probably you will probably fail the first time. You will have to think about, “Which area shall I start with? What kind of pilot projects should they put together? And along the journey, how I’m going to connect those pilot projects? So then little by little step by step, I’m going to build that AI talent capability that as an organization I need.” So that’s one thing is, “Do I have the talent?” as a question.
The other one which is really, really obvious is, “Do I have the data?” Because everyone knows that AI thrives in data. Obviously, not all of the AI techniques, but some of the techniques, especially when we talk about deep learning, you need tens of thousand of data to make those kind of techniques work. So do I have the data in size, but also in quality? Do we have the right quality of the data? Because again, regarding AI as a tool is garbage in, garbage out. Do we have the right data to feed by AI models? So those are the things that we should be thinking about at the beginning. And in addition to that, maybe we can go in more details later on, is the other principle around how do I develop and design and implement an ethical and bias AI model.
Debbie Forster:
So let’s talk about that. So let me make sure that I’ve captured it. I’ve got to get to the mindset where I have the knowledge, the talent, the capability within the team to be able to create great AI. In doing so, we have to change our mindset If we know how to use it well it is just another tool, okay? I don’t buy a hammer and run around saying, “Now somebody find me something to hit.” We know how to use our hammer and our saw and our screwdriver and know when to use it. So we’ll imagine then we have identified that problem. The data, we hear that again, I think a gold thread we have going through all of our podcasts and we get through all of tech these days is about data.
And I don’t think we can emphasize enough because we hear where there is bad AI on the basis of bad data, poor data, and there’s finding the cheapest way to scrape as much data is just the way, if you want to scrape what’s on the side of the road, I wouldn’t [inaudible 00:12:33]. So this is very much that garbage in, garbage out. But let’s then say, because I do think companies are beginning to get their head around us, but the ethics piece, so it’s not just what can we do, it’s understand what we mustn’t do or what we should be wary of. Talk me through that a bit.
Djamila Amimer:
Yeah. The ethics and the bias because they go together most of the time. I think it’s better explained through a concrete example. I think the best example will be if you’re a company in the recruitment space. So let’s say I have recruitment agency and I want to use AI to recruit and improve my business. So I need to understand, okay, so as a business context, what is the context of recruitment? So has recruitment changed over the years? Is the recruitment and the data profile of people of today similar to the 1940s of the 1940s or a hundred years ago? And the question is no, because back in the 1920s or 30s, if I take the example of a doctor, we will probably find male doctors compared to female doctors, which is completely different nowadays. Nowadays you find both alike. Similar for technical jobs, an engineering role back in the 1920s will probably be a man rather than a woman or a nurse role will be a woman rather than a man.
Debbie Forster:
Djamila, I think you’re very kind. We’d probably say the same thing about the 1980s.
Djamila Amimer:
Yes.
Debbie Forster:
But okay, yes. You’re being kind in recent years. But yes. And I couldn’t agree more because I think we have to go in when we’re looking at developing things. Actually, go looking for the bias in our data, look to where there may be problems and issues. We’ve seen the big scary ones like you say with recruitment, looking at identification around ethnicity, when we’re looking at crime data, et cetera, you have to really with that strong ethical eye go in testing for it, really digging in to see where those biases are going to be surfacing and then how can we build through that and how we can build the learning to start cleaning out that data or find yourself the cleanest data you can, but it is still that very important going in, not starry-eyed, just push the button. If we design it will be there, but looking through. Is there anything else around that bias piece that we should be aware of?
Djamila Amimer:
Yeah. So I think the most important bit is to understand your data. You cannot get data and then plug it into whatever tool. You have first to understand it. If you can’t understand it, then there is no point in building the AI model. You have to understand the characteristic of data, what kind of insight you may get from the data, whether there is a potential source of bias. For example, in the example of recruitment, we know if I take the 50 year ago data, we know that there will be bias. So I need to do something, I cannot just ignore that I don’t have to do something about it. But it doesn’t have to be historical.
So for example, even the companies who build a software recognition to recognize people. So if you build a platform to recognize people, so that’s people around all the planet, but then on the training you train only on white male pictures of white male, then it is a problem because it’s only going to recognize or based on what they have been trade on. So you need to think about is the data getting is having any bias and it’s the way I’m going to train the data is going to have a bias?
Debbie Forster:
It’s interesting also what you said about being able to understand our AI, explainable AI is becoming a very powerful concept. And one that isn’t just for good tech, but consumers, governments are beginning to ask for. Talk to me about your thoughts around explainable AI.
Djamila Amimer:
Absolutely. And I think obviously if you are a business and you operate in a highly compliant sector, for example, financial services or healthcare, you really need to understand what the algorithms are doing. So if I apply for a bank loan and the bank decline my loan, I have the right to go to the bank and claim, “Okay. Explain to me why you have declined my application.” And if you’re a bank who has been reckless and applied AI without understanding those methods and how your model is deriving those results, then you will be in a lot of problems and issues because suddenly you cannot explain to me why you refused Djamila her loan. So the way banks are addressing this is through what we call mitigation processes. So obviously, explainable AI is one big item.
I just need to clarify to the audience because a lot of people who talks about explainable AI and that AI is a black box. And I wanted to clarify this. AI is a black box only if you use the most sophisticated type of algorithm within AI. And we are mainly talking about deep learning. But if you use the less sophisticated of machine learning techniques, especially under the supervised family, without going in too much techie details, those techniques are pretty much explainable. So even the explainability issue, it’s not really applicable to the whole of AI, it’s really deep learning and some niche methods and techniques. But obviously, explainability is very important. So as a business owner who owns that model, you need to understand go step by step how this model is generating the output. What kind of correlation is making between this variable and this variable.
We know some of the correlation will be right, some of the correlation wouldn’t be true. For example, there have been lot of studies that linked for example, ice cream with the rate of divorce. We know that divorce has nothing to do with ice cream, but there is still a correlation between them. So you need to understand is the correlation a right one or just a dummy one? Another thing that banks are doing, it’s more than explainable AI. So in addition to explaining what they currently have, they’re having mitigation techniques. So they’re having another model that runs alongside the AI one, and they are monitoring the AI one. And there are some alerts in the system. So once an alert is being made, so something dodgy has been detected, then the secondary model will take over and then the AI model will be shut for that period until it gets fixed. So they’re having other models that run in parallel as a mitigation. They’re having signals alerts to tell them when things starts deviating from the way the model was supposed to work. So they’re trying to find mitigations in that sense.
Debbie Forster:
But I think that’s a key learning for us to take too if we’re in sectors that are not as compliance driven as financial services. Because I think if we understand as another of my podcast guests had talked about that millennials are now middle-aged and therefore key consumers in the market, we are getting a more and more tech savvy client partner user base out there, that having and wanting to understand that if this is good AI driving your product to a service. So adopting that mitigation mindset of how are we running things alongside to mitigate where things go wrong. I’d like to throw a question to you though. Let’s talk to some of our deeper techies in the audience. When we go into deep learning in relation to AI, is that sense of black box and therefore unexplainable AI inevitable or is that lazy thinking? What do we need to be thinking about in that spectrum when we start talking about deep learning in relation to AI.
Djamila Amimer:
Yeah. It’s not lazy thinking. So obviously, there is an issue around deep learning. Obviously, it depends how you set up the deep learning method and obviously the bigger it is, the more difficult is going to explain it. So we all heard of those models that use a hundred billions of parameters and thousands of layers. In this kind of things, it is very, very difficult to mitigate. But then there are things around that where you can then split. So if you have a bigger business problem or a business model, you split it into different pieces, yeah? And you put some protocols or some communications between the different compartments within that. So for example, if then one thing goes wrong in one thing, you would know that the wrong bit is happening in there and not in there. So one thing is to break down that complexity into smaller containable problems, which makes sense.
Another one is obviously, do the semi-supervised one. So you have a deep learning but then you have semi-supervised, so there is a human interaction that goes into the model and try to do that verification and modification judging how things are being done. So there are techniques you know can do, but the principle behind them is to make the complex less complex. So break down the complexity into smaller parts. So then it’s manageable.
Debbie Forster:
We could talk AI for days, but what I’d like to do is to let guests come outside just their own tower, ivory tower, keeping you in one lane. Let’s look to the future. Djamila, when you look at what is coming up on the horizon about anything within tech, what worries or annoys you the most?
Djamila Amimer:
Top one in my agenda in terms of concerns and worries, and we touched a little bit on is the ethics and the bias. I think for us in the AI community to make really a good step forward, a good advancement in this area. We cannot shy away from the ethics and the bias area. We need to find ways on how to tackle this. So that’s one thing. The second one is obviously the AI hype. It’s not helping because whenever there is a launch, for example the ChatGPT, and I’m not dismissing the capabilities of that or how good it is, but the way some companies are just in hurry to launch things and they’re not really given attention about the consequences of that because we really need to fix some of those issues if we really want to advance in this area.
Debbie Forster:
And we’ve watched this, haven’t we? For every hype curve that’s come through of everybody jumping in, realizing it is not magic and then coming back to solving real business problems in that way. Well, on a positive note, is there anything that’s coming up at the horizon that you’re excited about or you feel really positive about?
Djamila Amimer:
Yeah., so there are a lot of things. So one of them is symbolic AI. So I’m looking for ways, my thing that really excite me in this area is we need to make breakthroughs in terms of to make the current AI better for the future. And if we see what is currently lacking from ai, AI is lacking understanding and reasoning. It doesn’t understand and it doesn’t reason. The way you explain to people is the example of malaria and fever. So saying AI can find a correlation between malaria and fever, but AI would not understand that it is malaria that is causing the fever. So the causal effect, the causal reasoning is missing from AI. So I’m really look forward in any kind of breakthroughs or any kind of step stone in that direction. How can we build another type of AI that can have some reasoning capacity, that can have some causal reasoning skills. And symbolic AI could be one of them.
So it’s not the answer, but the answer is we have to be open. I think a lot of people in AI, or maybe let’s not exaggerate, maybe not a lot of people, but there are some people in AI who are close minded in their own piece of research. So we see, even the techniques, we talk about deep learning, we talk about the different machine learning techniques, most of the theory behind those techniques goes back to the 1940s, the 1950s. So along those days, even though we made breakthroughs in AI in terms of application and computer power, the theory behind it hasn’t really moved a lot. So we need to find ways to move the theory, the mathematical theory behind AI to a new area. And the way we do it is by opening our minds to other fields. So let’s not only focus, I know that deep learning is a big attractive area, but deep learning is not going to solve the causal reasoning for the future. So I want the AI community to open their mind and look at other fields.
Debbie Forster:
So that’s a shout-out, isn’t it? Right? To the community. Instead of just jumping on the hype and jumping in where everybody else is going, there are exciting new branches of thinking of AI that could offer the next big breakthrough, not just the next big theme, but the big breakthrough. But it’s going to take not just looking at application, but going back to those theoretical basics to get a different position for us to move forward.
Djamila Amimer:
Absolutely. And the key is to look into other fields and into other areas that we haven’t looked at and bring in all this together. That will be the key.
Debbie Forster:
Okay. So there you have it everyone. Your great open spaces. If you feel like you’re coming into tech and doing things and all the great things have been done, there’s your call to action to think about those new areas. Take the next breakthroughs. [inaudible 00:27:46] be able to talk about you and your [inaudible 00:27:48] in that you break it into that causal area to take the next big step. Listen, Djamila, thank you so much for doing this. It’s been great to hear what you’ve done, your journey and to get a sense of where AI could go. I really appreciate you coming in today.
Djamila Amimer:
Thank you so much, Debbie. Thanks for the invitation and it was a pleasure to talk to you about this interesting topic.
Debbie Forster:
Thank you for listening. If you’re a tech innovator and would like to appear as a guest on the show, email us now at [email protected]. And finally, thank you to the team of experts at Fox Agency who make this podcast happen. I’m Debbie Forster and you’ve been listening to the XTech Podcast.
Speaker 1:
Keep exploring the world of tech. Subscribe to our podcast and never miss an episode. To discover more opportunities for global B2B tech brands visit fox.agency today.